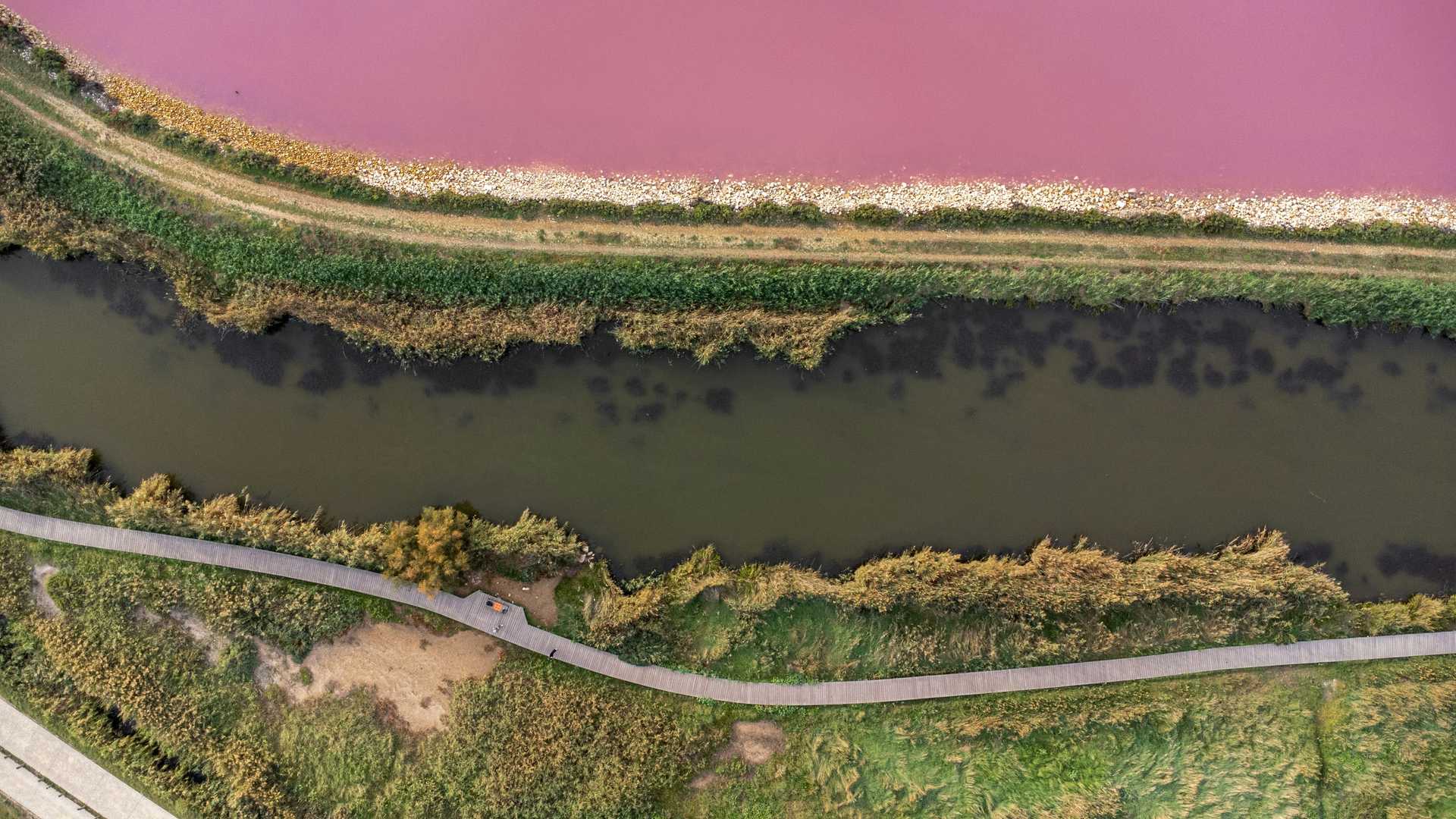
Predicting floods in ungauged watersheds
The majority of the world's watersheds are ungauged—they lack sensor infrastructure for measuring the flow of rivers directly. Absent historical streamflow data, the calibration of hydrological models for accurate flood prediction has been difficult. Prediction in ungauged basins was the decadal problem of the International Association of Hydrological Sciences from 2003 to 2012. Google's FloodHub model, however, uses Long Short-Term Memory (LSTM) recurrent neural networks to forecast river floods up to seven days in advance using publicly available data sources. Trained on weather and terrain data from 5,680 watersheds, totaling 152,259 years of data, was able to outperform the current state-of-the-art Global Flood Awareness System (GloFAS) in terms of precision, recall, and lead time for short-term (0-7 days) forecasts of extreme riverine events. The AI model, developed by Google Research, now powers an operational early warning system that provides free, real-time forecasts in over 80 countries.
Tools like this force governments to up their adaptation game. When the U.S. Embassy in Beijing began to tweet air quality readings in 2008, it forced the Chinese government to address urban air pollution. Similarly, as open AI models for predicting extreme hazard events can be trained from remotely sensed data, it will force nations and cities to address climate hazards more swiftly and directly, enhancing flood prediction and early warning systems that can protect millions.