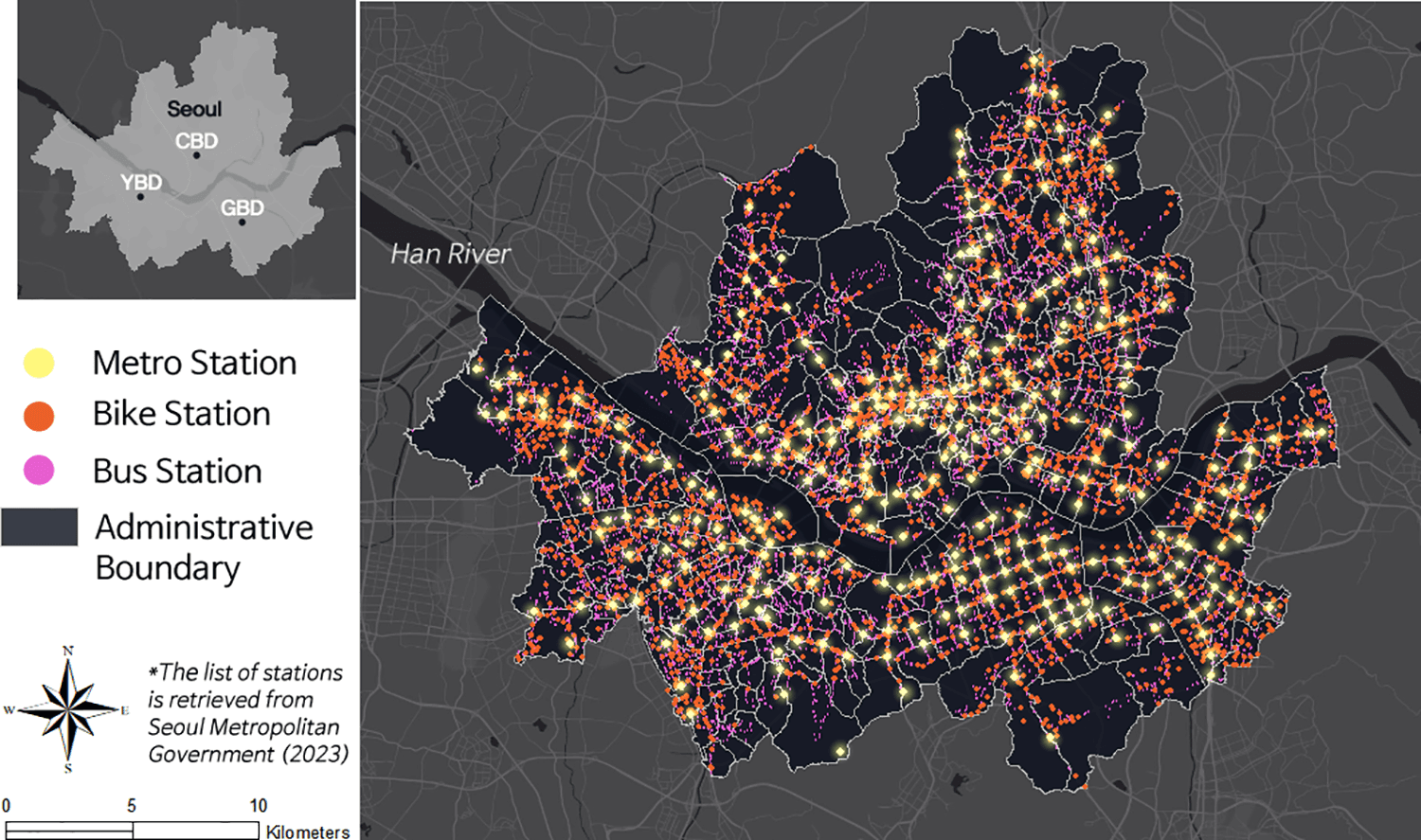
Modeling transit deserts in real-time
Transit deserts - areas where public transportation service levels fail to meet the mobility needs of the population - have become a growing concern in urban planning. Traditional studies of these areas are largely descriptive and static, identifying locations where transit demand exceeds supply at a single point in time, without exploring underlying causes. This study of Seoul, South Korea used advanced machine learning techniques including a random forest model to forecast the presence of transit deserts based on key factors including density, distance to transit, diversity in the built environment, actual population movements over time, and sociodemographic characteristics. Paired with an interactive dashboard, the model updates transportation equity and accessibility analysis results as users adjust the threshold values for their neighborhoods.
As user-friendly tools for interacting with complex models for classifying vulnerable urban zones become more widespread, they will transform how AI models are used in planning. In adaptation planning, this means models are becoming central to facilitating community dialogue and participatory processes. Rather than experts simply producing one-time analyses, planners can now use these tools to simulate different scenarios in real-time during community meetings, test various intervention strategies, and visualize their potential impacts. Additionally, when city residents have access to such tools, they can independently explore different planning scenarios, better understand the trade-offs involved in transportation decisions, and more meaningfully participate in the planning process, ultimately leading to better-informed and more widely supported transportation solutions.