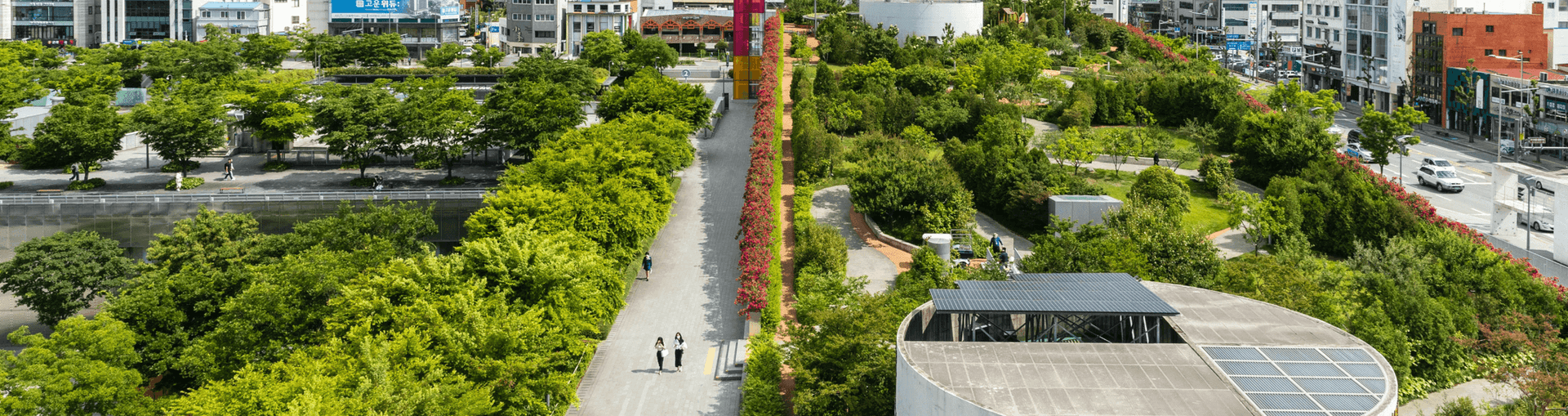
Hyper-local, multi-hazard exposure models
The Urban Systems Lab (USL) is developing ClimateIQ, an integrated multi-hazard modeling environment for cities facing elevated risks from extreme weather due to climate change. Funded by a $5 million grant from Google.org, the tool will provide high-resolution urban heat and extreme flood hazard exposure predictions drawn from two hyper-local physics-based models. Machine learning models will be trained to reproduce the outputs of the physics-based models, speeding up climate hazard simulation time. To make hazard information available to users, the project will partner with startup ClimaSens to provide a user-friendly dashboard and APIs, along with ongoing technical support and data management services to ensure successful implementation and maintenance. Pairing the outputs of climate models with detailed data and vulnerability, the tool will allow cities to assess exposure from multiple and cascading impacts of extreme weather on infrastructure and vulnerable populations. The project aims to work with up a dozen cities cities around the globe facing a broad array of climate change challenges from sea level rise to desertification. A particular focus will be on less-resourced or smaller cities that lack the climate analysis and modeling capabilities of larger cities, with the scalable solution designed to grow alongside cities' needs and capabilities. The advent of tools for faster, hyper-local, multi-hazard exposure assessment to can provide cities with better information for decision-making around both resilience investments and operations and response to weather-related events, boosting both short-term and long-term resilience. And by democratizing access to this data, they can level the playing field for cities lacking resources for comprehensive climate risk assessments.