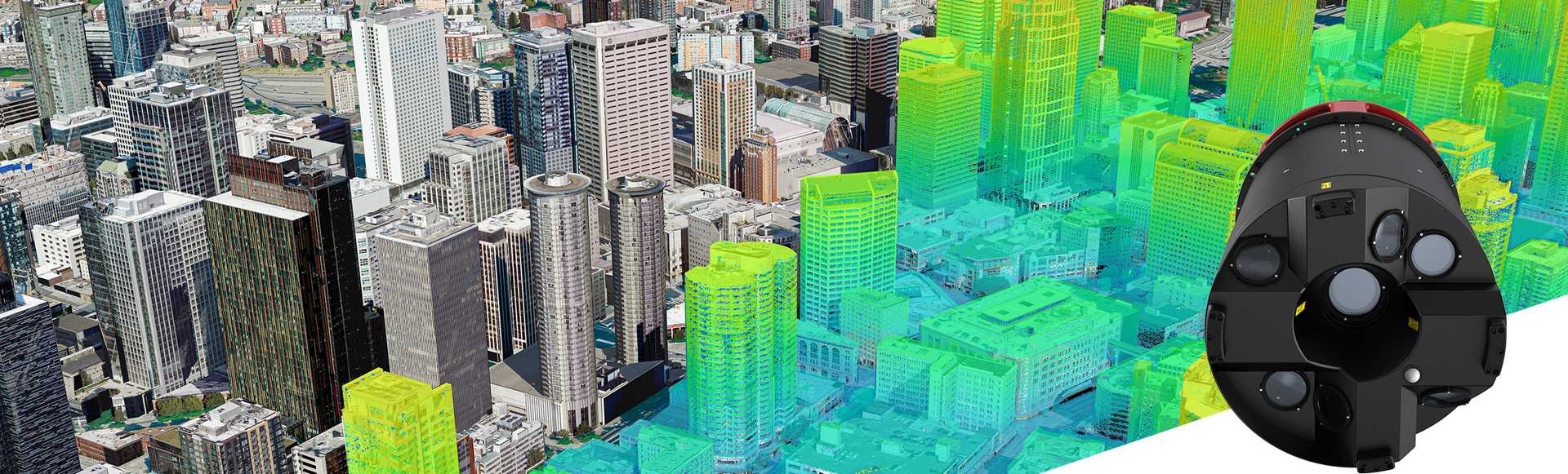
Big models for tiny devices
Current AI approaches are resource-intensive, often relying on large computing infrastructures and cloud-based services, which are impractical in remote areas with limited memory, compute power, energy, and network connectivity. This is especially true of generative AI—not only for initial training of massive foundation models with many billions of parameters, but also inference—or the use of such models to draw insights from new data. However, new approaches to adapt generative AI to resource-constrained environments, common in urban climate adaptation applications, involve development of tiny, offline models suitable for edge devices, smaller computers deployed within urban infrastructure, vehicles, and mobile devices. Model compression techniques like pruning, quantization, and knowledge distillation are being used reduce model size and make them suitable for edge devices.
As low-resource AI models suitable for edge devices with low power and connectivity requirements become more widely available and capable, they will make it easier to embed sophisticated AI capabilities within urban infrastructure networks and structures, enabling new climate adaptation innovations that bolster urban resilience.